The next 18 months will separate AI drug discovery’s pioneers from its tourists. Here’s where the rubber meets the road—and where most teams will skid out.
1. Generative Models vs. Wet-Lab Validation
Current Reality: AI platforms like GALILEO™ and AtomNet generate thousands of novel compounds in silico, with hit rates up to 70% in preclinical studies6. But wet-lab validation remains the bottleneck – only 14% of AI-predicted molecules show clinical viability4.
False Comfort: Assuming in-silico accuracy translates to biological relevance.
Risk: Overfitting to synthetic datasets creates “paper drugs” that fail in vivo. Atomwise’s recent TYK2 inhibitor success relied on iterative wet-lab feedback to refine AI predictions6.
Signal to Watch: Partnerships between AI-first startups (e.g., Unlearn1) and CROs with high-throughput screening infrastructure.
Our Move: Mandating parallel wet-lab testing for every AI-generated batch – no exceptions.
“AI drug discovery’s make-or-break factors by 2026: Wet-lab validation loops, FDA adaptability, hybrid talent, interpretable tools, and avoiding target overfitting. Teams ignoring these will join the 90% of AI pharma startups that fail to exit preclinical.”
2. FDA’s Regulatory Tightrope Walk
Current Reality: Zero FDA-approved AI-discovered drugs exist. The agency’s 2024 draft guidance on AI/ML in drug development lacks concrete validation benchmarks25.
False Comfort: “The FDA will adapt” is a dangerous mantra. Current review panels lack AI/ML expertise, and explainability hurdles persist2.
Risk: Delayed approvals for AI-driven candidates (see Insilico’s quantum-hybrid oncology pipeline3).
Signal to Watch: First-mover advantage for teams engaging FDA early via Breakthrough Device pathways.
Our Move: Co-developing audit trails with regulators – every AI decision node is documentable.
3. The Talent Chasm: Coders ≠ Biologists
Current Reality: Big Tech engineers dominate AI drug discovery teams, but 53% of failed AI pharma projects trace back to biological misinterpretations4.
False Comfort: Hiring more computational biologists. Reality: True interdisciplinary thinkers—those fluent in PyTorch and PCR – are rare.
Risk: Misaligned model architectures (e.g. overprioritizing binding affinity over ADME properties).
Signal to Watch: Surge in “hybrid” roles (e.g., “Quantum Biology Engineer” postings at Model Medicines3).
Our Move: Dual-track training: forcing engineers to shadow lab techs, and biologists to hack Jupyter notebooks.
4. Interpretability Tools for Skeptical Biologists
Current Reality: Biologists distrust black-box AI. Only 22% of pharma teams use AI outputs without manual review2.
False Comfort: “SHAP values and attention maps are enough.” They’re not – biological systems demand causal reasoning.
Risk: Reversion to traditional methods by risk-averse CSOs.
Signal to Watch: Tools like Unlearn’s digital twin generators, which map AI predictions to known clinical endpoints1.
Our Move: Building “glass-box” models where every prediction links to published pathways (e.g., KEGG, Reactome).
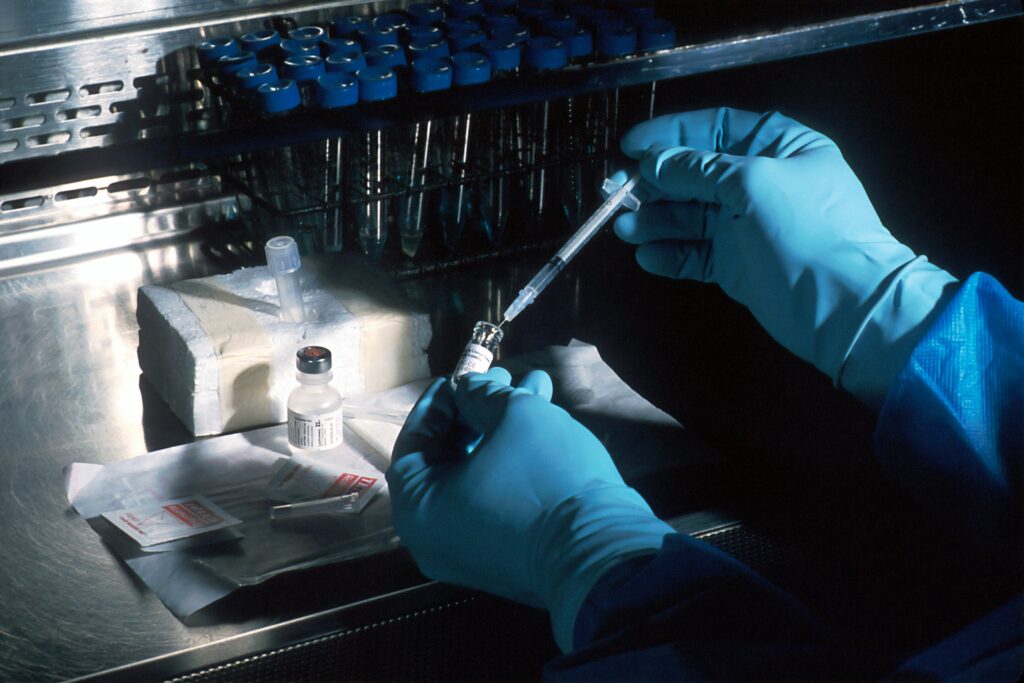
5. Target Overfitting: Speed ≠ Impact
Current Reality: 68% of AI pipelines target kinase inhibitors or GPCRs – low-hanging fruit with crowded IP6.
False Comfort: “We’ll pivot to novel targets later.” Reality: Legacy data biases trap models in familiar territory.
Risk: A glut of “me-too” AI drugs with marginal differentiation.
Signal to Watch: Insilico’s quantum-classical models targeting previously “undruggable” proteins3.
Our Move: Penalizing teams for easy targets – literally. Bonus structures tied to first-in-class nominations.
The Bottom Line: AI drug discovery isn’t a tech stack – it’s a new biology paradigm. The next 18 months will ruthlessly expose who’s building scaffolds and who’s painting facades. Watch the wet-lab validations, regulatory dialogues, and target diversity metrics. That’s where the real signal lives.
If you are looking for the winning team to take you to that next level, get in touch.